The DP-600 certification exam validates your ability to implement analytics solutions using Microsoft Fabric, certifying you as a Fabric Analytics Engineer. 🧑🎓
The exam covers essential skills and key components such as warehouses, lakehouses, data pipelines, dataflows, and semantic models. The key skills required for the DP-600 exam include:
- Maintain a data analytics solution (25–30%)
- Prepare data (45–50%)
- Implement and manage semantic models (25–30%)
This page provides a comprehensive overview of the key skills covered in the DP-600 exam. We provide step-by-step tutorials to each exam topic, helping you to prepare for the DP-600 exam and master the skills needed to become a certified Fabric Analytics Engineer. 🚀
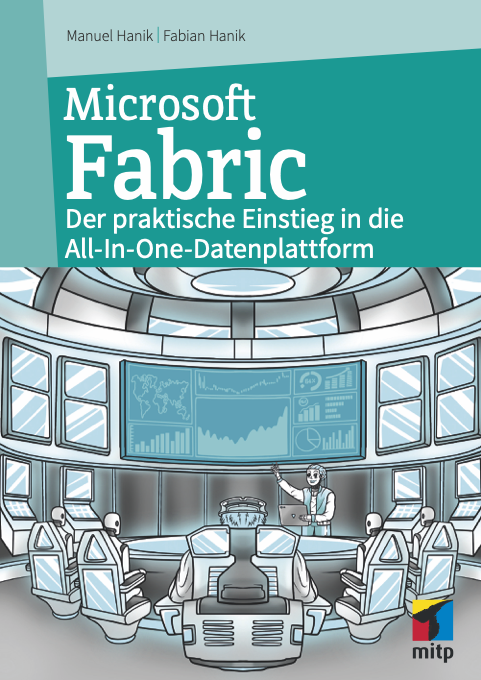
Microsoft Fabric Book
We are delighted to publish a hands-on guide to implementing end-to-end data projects in Microsoft Fabric. This hands-on book walks you through the key components and functionalities of Microsoft Fabric and invites you to actively follow the steps yourself. Numerous visual elements are used to make learning even clearer. The explanations are illustrated by a fictional story about a futuristic data factory that symbolizes Microsoft Fabric.
View on AmazonMaintain a data analytics solution (25–30%)
Implement security and governance
- Implement workspace-level access controls
- Implement item-level access controls
- Implement row-level access control
- Implement column-level access control
- Implement object-level access control
- Implement file-level access control
- Apply sensitivity labels to items
- Endorse items
Maintain the analytics development lifecycle
- Configure version control for a workspace
- Create and manage a Power BI Desktop project (.pbip)
- Create and configure deployment pipelines
- Perform impact analysis of downstream dependencies from lakehouses, data warehouses, dataflows, and semantic models
- Deploy and manage semantic models by using the XMLA endpoint
- Create and update reusable assets, including Power BI template (.pbit) files, Power BI data source (.pbids) files, and shared semantic models
Prepare data (45–50%)
Get data
- Create a data connection
- Discover data by using OneLake data hub and real-time hub
- Ingest or access data as needed
- Choose between a lakehouse, warehouse, or eventhouse
- Implement OneLake integration for eventhouse and semantic models
Transform data
- Create views, functions, and stored procedures
- Enrich data by adding new columns or tables
- Implement a star schema for a lakehouse or warehouse
- Denormalize data
- Aggregate data
- Merge or join data
- Identify and resolve duplicate data, missing data, or null values
- Convert column data types
- Filter data
Query and analyze data
- Select, filter, and aggregate data by using the Visual Query Editor
- Select, filter, and aggregate data by using SQL
- Select, filter, and aggregate data by using KQL
Implement and manage semantic models (25–30%)
Design and build semantic models
- Choose a storage mode
- Implement a star schema for a semantic model
- Implement relationships, such as bridge tables and many-to-many relationships
- Write calculations that use DAX variables and functions, such as iterators, table filtering, windowing, and information functions
- Implement calculation groups, dynamic format strings, and field parameters
- Identify use cases for and configure large semantic model storage format
- Design and build composite models
Optimize enterprise-scale semantic models
- Implement performance improvements in queries and report visuals
- Improve DAX performance
- Configure Direct Lake, including default fallback and refresh behavior
- Implement incremental refresh for semantic models